Two people have emailed me this story (one via Stuff, one via the Herald) about the DNA ancestry of Oriini Kaipara, a TV presenter:
An analysis of the DNA of Oriini Kaipara, 33, has shown that – despite her having both Maori and Pakeha ancestry – her genes only contain Maori DNA. That makes her, in her own words, a “full-blooded Maori”.
Culturally, people identify as Maori through their whakapapa, while legally a person is defined as Maori if they are of Maori descent, even through one long-distant ancestor.
However, the intermingling of different ethnicities in New Zealand over the past 200 years means all Maori people are thought to have some non-Maori ancestry, so would not be expected to have 100 per cent Maori DNA.
It seems strange that someone could have an ancestor from whom they got no DNA, but while most ‘ancestry and genetics’ news stories are completely bogus, this one probably isn’t.
Ignoring the X and Y chromosomes to start with, you have 22 chromosomes from your mother and 22 from your father (except for some rare cases such as people with Down syndrome, who have an extra copy of one of them, usually from their mothers). Each of your maternal chromosomes is a combination of DNA from your mother’s father and mother’s mother, in chunks averaging about 1/4 chromosome long. Each of your paternal chromosomes is a combination of DNA from your father’s father and father’s mother, in chunks averaging about 1/4 chromosome long. So, on average, you have 1/4 of your DNA from each grandparent, but it’s random. You might have only tiny chunks from one grandparent and almost 50% from another.
As we go back further, after N generations you have 2N direct ancestors, but the chunks of DNA being inherited are about 1/2N chromosomes long. So, going back 10 generations you have 1024 ancestors and you’re inheriting DNA chunks about 1/20th of a chromosome long. But with 22 pairs of chromosomes, that only allows you to fit in chunks from 20×2×22=880 of your great8-grandparents. So, you almost certainly have DNA from all your grandparents, and very likely from all your great-grandparents, but it’s unlikely you have DNA from all your ancestors ten generations back, and the proportion you have DNA from goes down and down the further back you go. Europeans in NZ don’t go all that far back, so the probability is pretty high for any given European ancestor of a modern Māori, but it’s not 100%.
In modern New Zealand, most Māori will have more non-Māori ancestors than Ms Kaipara does, and most people with only two non-Māori ancestors will have inherited DNA from at least one of them, so it would be unusual for someone to have no non-Māori DNA, but certainly not impossible.
The next question is how the genetic testing people can know which DNA came from Māori ancestors. The DNA bases that end up in a saliva sample are synthesised in your body from the food you eat: they don’t come with little labels saying which ancestor’s DNA they are copies of. One adenine base looks just like any other. The approach to this problem is statistical: there are many, many positions in the DNA sequence where particular variations are more common in one part of the world than in others. Some of these are well known because of what they do, but those are a tiny minority; nearly all of them are unimportant copying errors. In any case, two people who share the variant probably got it from the same distant ancestor, so if you collect enough DNA variants from enough people around the world, you can tell with surprising reliability where people’s ancestors came from.
Here’s a picture from research in the USA, showing three genetic summaries for people identifying with various Hispanic/Latinx groups:
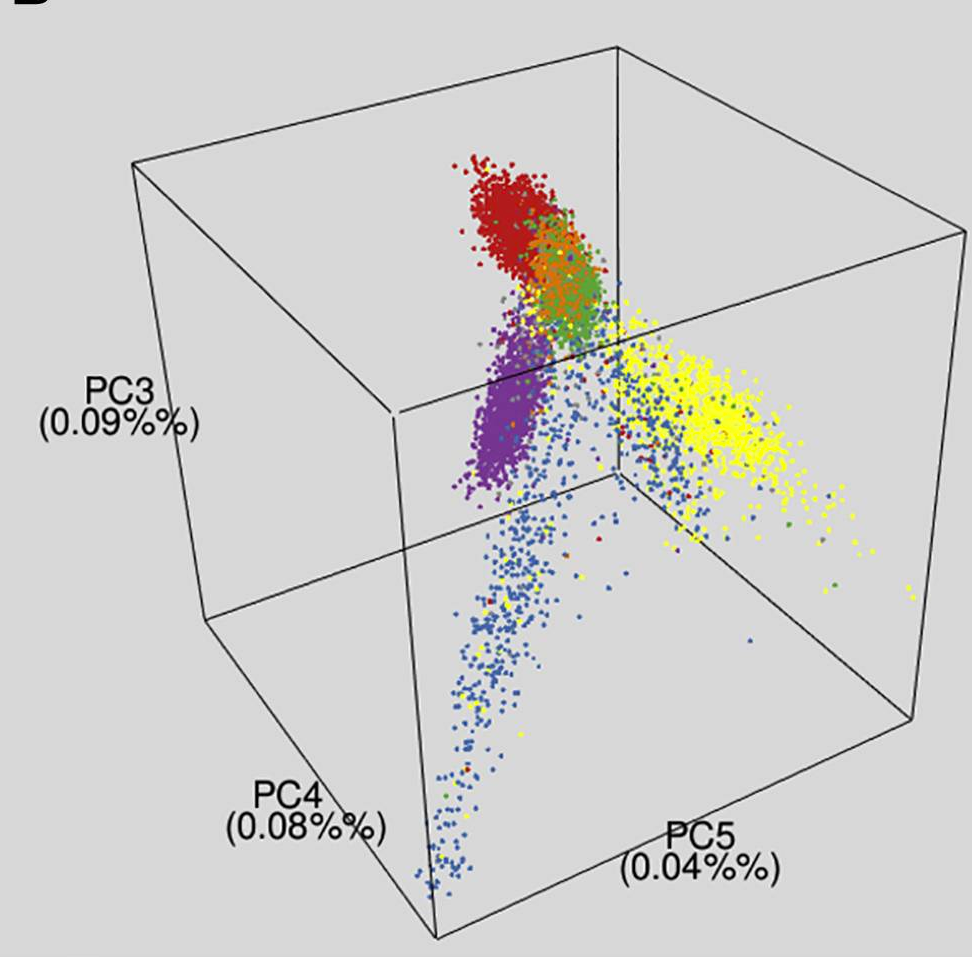
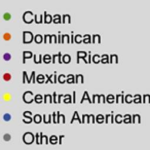
There’s pretty clear separation: in this sample you can tell quite a lot about a typical person’s ancestry from their genes. No single genetic variant will tell you much, but thousands or millions of them together tell you a lot. In this example, the three summaries correspond roughly to amounts of ancestry from the Americas before Columbus, from Europe, and from western Africa via the slave trade. are the most important variation after the first three summaries giving basic continental ancestry are taken out.
The test used by ancestry.com measures 700,000 DNA variants, which is a respectable number. It’s probably a bit short on markers for Polynesian ancestry, because there hasn’t been much genetic study of Polynesians. It will be very short on markers that distinguish Māori from other people with Polynesian ancestry, but in this example, family history was enough to make that unnecessary. So, it’s plausible that some Māori have little or no non-Māori DNA, and it’s plausible that ancestry.com could determine that with reasonable reliability: the story is making a claim that has some content and could very well be true. As the story says, the result doesn’t actually matter much, but it is interesting.
Without Ms Kaipara’s family history, just using genetic data, the video clip says her Polynesian ancestry was estimated as between 93% and 100%: there’s quite a bit of uncertainty. For someone with a less clearly known family history, or from somewhere that mixing of populations happened longer ago than two centuries, the test will be less informative, but will still give some general information about what parts of the world your ancestors may have come from. You might still want to know.
What this story should make you concerned about, though, is other news stories talking about someone’s descent from, say, Genghis Khan. If Ms Kaipara can have recent ancestors whose DNA she doesn’t appear to carry, how can claims from 1000 years in the past be credible? And indeed they aren’t. As you go back further and further in time, you have more and more ancestors. By the time of Genghis Khan, there would be tens of billions of them. Obviously there must be huge overlap, but that still allows you to be descended from a lot of people. Pretty much everyone in Europe and Asia has Genghis Khan as an ancestor; a fraction of them carry DNA descended from his; and a tiny fraction of these have copies of his Y chromosome. The test results that more often make headlines are the last sort, which are pretty meaningless.